Artificial intelligence (AI) is revolutionizing the way we approach forecasting in both baseball and trading, offering insights that were previously unattainable. In baseball, AI algorithms analyze vast datasets, including player performance, weather conditions, and historical game outcomes, to predict future game results and player performance. Teams and coaches use these predictions to make strategic decisions, optimizing line-ups and game strategies to enhance their chances of winning. Similarly, in the trading world, AI plays a crucial role in analyzing market data, identifying patterns, and predicting future price movements. Traders and financial institutions leverage AI-driven models to make informed decisions, manage risks, and capitalize on market opportunities.
The application of AI in trading is exemplified by the Exness broker, which utilizes advanced AI technologies to provide its clients with cutting-edge market analysis and forecasting tools. By integrating AI into their platforms, Exness and other brokers can offer traders real-time insights, predictive analytics, and automated trading strategies, significantly enhancing their ability to make profitable trades. In both baseball and trading, AI’s predictive capabilities are not just about anticipating the future; they also provide a strategic edge, enabling professionals to make more informed decisions, reduce risks, and maximize performance. Whether on the baseball field or in the financial markets, AI is a game-changer, transforming data into actionable intelligence and redefining the landscape of forecasting.
Overview of Artificial Intelligence (AI)
Artificial Intelligence (AI) represents a significant leap in the evolution of technology, offering machines the capability to process information, make decisions, and perform tasks that traditionally require human intelligence. This field encompasses a variety of techniques and disciplines aimed at creating systems that can learn, reason, perceive, infer, communicate, and act in the physical world. The essence of AI is not just in programming machines to execute tasks but in enabling them to learn and adapt from experience, enhancing their performance over time.
Machine Learning (ML)
One of the pivotal components of AI is machine learning (ML), a subset where algorithms are designed to analyze data, learn from it, and make predictions or decisions. Unlike traditional programming, where actions are explicitly coded, ML enables systems to evolve their understanding and improve their accuracy without being explicitly programmed to do so. This adaptability is at the heart of many modern AI applications, from recommendation systems on streaming platforms to predictive models in finance and healthcare.
Natural Language Processing (NLP)
Natural Language Processing (NLP) is another crucial area of AI, focusing on the interaction between computers and humans using natural language. The goal is to enable machines to understand, interpret, and generate human language in a way that is both meaningful and contextually relevant. This capability is fundamental to developing effective AI assistants, translating languages, and extracting insights from text data.
Robotics
In robotics, AI is instrumental in creating autonomous machines that can perform tasks in dynamic and complex environments. These robots, equipped with AI, can analyze their surroundings, make decisions, and execute actions with a degree of independence akin to living creatures. This integration of AI transforms robots from mere programmable entities to intelligent agents capable of learning and adapting.
Computer Vision
Computer vision, another domain of AI, empowers machines to interpret and make decisions based on visual data. This field has made significant strides in enabling technologies like facial recognition, image analysis, and autonomous vehicles, where machines must perceive and understand their environment to make informed decisions.
Expert Systems
Expert systems in AI mimic the decision-making abilities of human experts, providing specialized guidance and decisions in fields like medicine, engineering, and finance. These systems leverage AI’s reasoning capabilities to assess complex scenarios and offer recommendations or decisions that would typically require a human expert’s insight.
As AI continues to evolve, its impact is being felt across various sectors, driving innovation and offering new solutions to age-old problems. The potential of AI is vast, with its applications ranging from the mundane to the groundbreaking, continually pushing the boundaries of what technology can achieve.
Introduction of AI in Baseball Forecasting
The introduction of Artificial Intelligence (AI) in baseball forecasting marks a transformative era in the sport, leveraging advanced technologies to enhance strategic decision-making and performance analysis. AI in baseball combines the power of machine learning, data analytics, and predictive modeling to analyze vast amounts of data that the sport generates, offering insights that were previously inaccessible or too complex to decipher manually.
AI-driven forecasting in baseball involves the analysis of player statistics, game outcomes, environmental conditions, and other relevant data points to predict future game results, player performances, and even injury risks. These predictive insights help teams optimize their strategies, from lineup selections to in-game tactics, providing a competitive edge that is grounded in data-driven analysis.
Machine learning models in baseball forecasting learn from historical data, identifying patterns and correlations that human analysts might overlook. For instance, AI can predict how a player’s performance might vary under different conditions or how they might fare against specific opponents. This level of analysis provides a granular understanding of the game, enabling teams to tailor their strategies to maximize their chances of success.
Moreover, AI in baseball is not just about forecasting outcomes but also about enhancing player development and injury prevention. By analyzing data from wearable devices and training sessions, AI systems can recommend personalized training and recovery programs, reducing injury risks and optimizing player performance over time.
The integration of AI in baseball forecasting signifies a broader trend in sports analytics, where data and technology converge to elevate the game’s understanding and execution. As these AI systems continue to evolve and learn, their predictions and insights will become increasingly sophisticated, further revolutionizing how decisions are made in baseball.
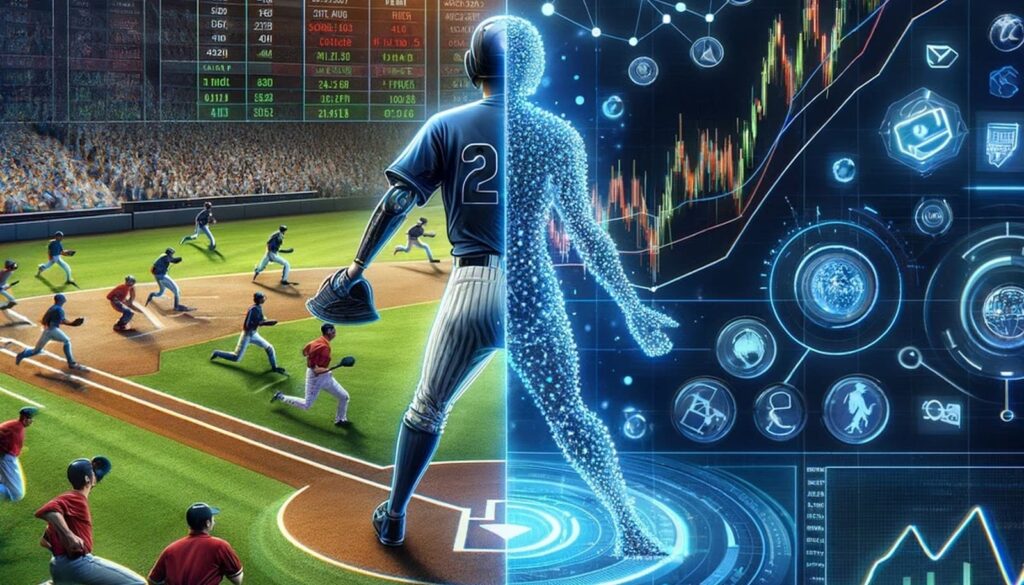
Differences Between Baseball and Trading Forecasting With AI
The application of Artificial Intelligence (AI) in forecasting has made significant strides in both baseball and trading, yet the context, objectives, and nuances in these fields lead to distinct differences in how AI is deployed and the outcomes it aims to achieve.
- Objective Differences:
- In baseball forecasting, AI primarily aims to enhance team performance, predict game outcomes, strategize player placements, and optimize training regimes. The focus is on competitive advantage, player performance metrics, and injury prevention.
- In trading forecasting, AI seeks to predict market movements, identify trading opportunities, and manage financial risk. The objective is financial gain through informed decision-making, risk assessment, and market analysis.
- Data Nature and Volume:
- Baseball AI systems analyze player statistics, game results, physical and environmental conditions, and more. The data is structured, time-series based, and relatively finite compared to financial markets.
- Trading AI systems deal with vast amounts of high-velocity, often real-time financial data, including price changes, trading volumes, economic indicators, and news feeds. The data is more volatile and unstructured, necessitating sophisticated models to handle noise and rapid changes.
- Model Complexity and Predictability:
- Baseball forecasting involves more stable and predictable variables, where past performance and statistical analysis can offer strong indicators of future outcomes. AI models here may not need to adapt as rapidly as in trading.
- Trading models must contend with market efficiency and the inherently unpredictable nature of financial markets, where countless variables interact in complex ways. AI systems in trading often employ more complex, adaptive algorithms capable of real-time learning and adjustment to market dynamics.
- Impact of Unpredictability:
- In baseball, unpredictability comes from player conditions, unexpected plays, or environmental factors, but the game’s structure limits randomness. AI systems can reasonably account for such variables over time.
- In trading, unpredictability is a fundamental characteristic, with market sentiment, geopolitical events, and other externalities playing significant roles. AI systems must continuously adapt to new information, making forecasting inherently more speculative and risk-prone.
- Feedback Loops and Adaptation:
- AI in baseball benefits from relatively clear, immediate feedback through game outcomes and player performance metrics, allowing for iterative refinement of strategies.
- In trading, feedback loops are rapid and can be extreme, requiring AI systems to adapt quickly to avoid significant financial losses. AI strategies in trading must constantly evolve due to the adaptive nature of markets where today’s successful strategy might fail tomorrow due to market participants’ counter-actions.
In essence, while both baseball and trading use AI to forecast and strategize, the underlying dynamics, objectives, and data environments in each field necessitate different approaches, model complexities, and adaptability in AI applications.
Definition of AI in Trading Forecasting
AI in trading forecasting refers to the application of artificial intelligence technologies to predict future movements in financial markets. This involves using algorithms and machine learning models to analyze historical data and identify patterns that can suggest future market trends. AI systems in trading are designed to process vast amounts of data at high speeds, including price movements, trading volumes, economic indicators, and news events, to make informed predictions about future market behavior.
These AI models can adapt to new data and learn from their successes and failures, continually refining their predictions. In trading forecasting, AI can be used for various tasks, such as predicting stock prices, identifying potential investment opportunities, assessing risk, and executing trades at optimal times. The goal is to outperform traditional, human-driven trading strategies by leveraging the speed, volume, and complexity of data processing that AI provides.
AI in trading forecasting not only analyzes structured data like financial indicators and market prices but also unstructured data such as news articles, social media posts, and economic reports to gauge market sentiment and potential impacts on trading. By integrating diverse data sources and continually learning from market dynamics, AI in trading aims to provide more accurate, timely, and actionable forecasts, thereby enabling traders and financial institutions to make more informed, data-driven decisions.
Recap of the Significance of AI in Forecasting for Baseball and Trading
The significance of AI in forecasting for both baseball and trading lies in its ability to process vast datasets, identify patterns, and make predictive insights that surpass the capabilities of traditional analysis methods. In each domain, AI leverages specific data types and models to cater to unique objectives and challenges, providing substantial benefits:
Baseball Forecasting:
- Enhanced Performance Analysis: AI analyzes player and team performance data to provide deep insights that help in improving player strategies and team compositions.
- Game Outcome Predictions: By evaluating historical and current data, AI helps forecast game results, aiding teams in strategizing more effectively.
- Injury Prevention and Player Fitness: AI models can predict potential injury risks and offer recommendations for training and recovery, thereby optimizing player health and career longevity.
- Talent Scouting: AI can assist in identifying promising players by analyzing performance data across various levels, helping teams make informed recruitment decisions.
Trading Forecasting:
- Market Trend Analysis: AI processes historical and real-time market data to identify trends, helping traders make informed decisions on buying, selling, or holding assets.
- Risk Management: By predicting market volatility and identifying potential risks, AI enables traders and institutions to devise strategies that minimize losses and optimize gains.
- Algorithmic Trading: AI algorithms can execute trades at superhuman speeds and volumes, capitalizing on fleeting market opportunities that humans cannot process quickly enough.
- Sentiment Analysis: AI evaluates unstructured data like news and social media to gauge market sentiment, providing traders insights into potential market movements influenced by public perception.
In both fields, AI’s ability to learn from data and adapt to new information means that its forecasts and recommendations become continually refined over time. While in baseball, the focus is on enhancing competitive performance and player management, in trading, it’s about maximizing financial returns and minimizing risks. Despite these different applications, the underlying value of AI in both domains is its capacity to transform vast, complex datasets into actionable insights, thereby enabling more informed decision-making and strategic planning.
Conclusion
The integration of Artificial Intelligence (AI) into baseball and trading forecasting represents a monumental shift in how data is analyzed and utilized in decision-making processes. In baseball, AI enhances strategic planning, player performance optimization, injury prevention, and talent scouting, fundamentally transforming the sport’s analytical landscape. For trading, AI revolutionizes market analysis, risk management, and trading execution, providing a substantial edge in the fast-paced, data-intensive financial markets.
The core strength of AI in both domains lies in its ability to digest massive datasets, uncover hidden patterns, and adapt to new information, delivering insights and forecasts that are beyond the reach of traditional methods. While the specific applications and outcomes differ in baseball and trading, the underlying impact of AI is to empower organizations and individuals with predictive insights that drive smarter, data-driven decisions.
As AI technologies continue to evolve, their potential in forecasting across various fields will likely expand, offering even more sophisticated tools for analysis and decision-making. The continued adoption and advancement of AI in baseball and trading are set to redefine the future landscapes of these fields, highlighting the transformative power of AI in harnessing data to inform and enhance human decision-making.